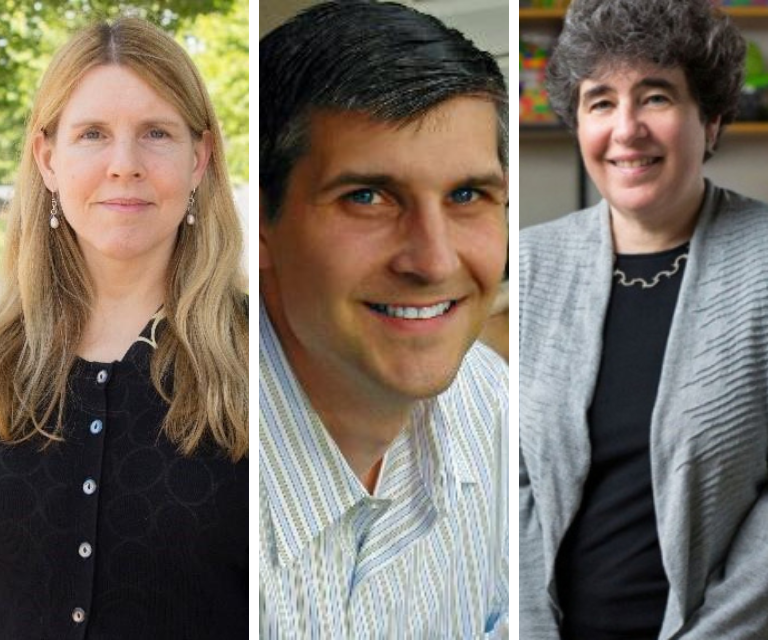
Using Artificial Intelligence to Evaluate Classroom Discussion Quality
University of Pittsburgh School of Education faculty members Rip Correnti and Lindsay Clare Matsumura are teaming up with Diane Litman from the Pitt School of Computing and Information to create a web-based application that uses artificial intelligence (AI) to analyze the quality of classroom discussion.
The project was selected for a Catalyst Prize in the 2021-2022 Learning Engineering Tools Competition, a global search for promising new education ideas, technologies, and platforms. The Pitt team was one of 30 winners selected from over 800 entries from 60 countries.
The AI application will analyze transcripts of classroom discussions using natural language processing and machine learning methods. The transcripts will be automatically scored based on such factors as how students are asked to explain their thinking, how teachers connect students’ contributions to each other, and how students collaborate with one another.
“Classroom discussions are essential to student learning across the content areas,” says Matsumura, a Pitt Education professor and a senior scientist in the Learning Research and Development Center (LRDC). “On the teacher’s part, it’s difficult to learn how to do these types of discussions, but they form the heart of the most ambitious teaching reforms.”
“We like to look at discourse in the classroom to get a gauge on how we can operationalize measures of instructional quality,” says Correnti, a Pitt Education associate professor and an LRDC research scientist. “We’re trying to expand what we can do in the AI sphere to better understand instructional quality and think about interventions that build off of that.”
Correnti and Matsumura previously worked on an automated writing evaluation system with Litman, a professor of computer science and an LRDC senior scientist. That project, which scored student essays and provided students with automated feedback, laid groundwork for this new classroom discussion project.
“In the AI in education and natural language processing community, this type of data is difficult to work with,” says Litman. “There are a few other efforts in the country where people are starting to take baby steps into assessing classroom discourse.”
Using a measurement scheme previously developed by Correnti and Matsumura, the team will start building the tool using transcripts of fourth- and fifth-grade classroom discussions and students’ reading outcomes.
“One of the issues in research is that collecting and scoring classroom discussions is a very costly and time-consuming endeavor, which is why these kinds of studies are usually limited to small numbers of classrooms. This limits the inferences you can make from the data,” says Matsumura. “We endeavor to develop a tool that will provide a more accessible way to advance learning science research by enabling in-depth analyses of classroom discussions in large numbers of classrooms.”
The application will be developed first as a tool for learning scientists and, eventually, the team would like to scale it up for classroom teachers to analyze their own discussions.
“We could open up avenues for researchers to examine discourse and think more deeply about how to improve instructional quality,” says Correnti. “As we perfect the tool and make it more usable for teachers in the classroom, it could help teachers reflect on their own discourse and identify interventions.”
The team also hopes the tool will shed light on inequities in students’ learning opportunities.
“It is so difficult to get multidimensional measures of the quality of classroom talk,” says Matsumura. “We will be able to have a better sense of the classroom learning opportunities for students who have been traditionally underserved in the school system.”
“Our work on instructional quality shows us that we need to foster ways for teachers to invite students to do the thinking and reasoning,” says Correnti. “Classroom discussions can help students learn to feel agency and speak up when they see injustice in the world. That can make them agents for making things more equitable in the future.”
Learn More
The University of Pittsburgh Learning Research and Development Center is dedicated to advancing knowledge about the science of learning and improving education practice and opportunities for all. Learn more about how the center advances the science of learning.